Data quality in CRM is not just a technical issue — it’s proper business process definition and user adherence to agreed-upon standards.
Erroneous and incomplete CRM data costs time and money. Good data can help secure new business and retain existing customers.
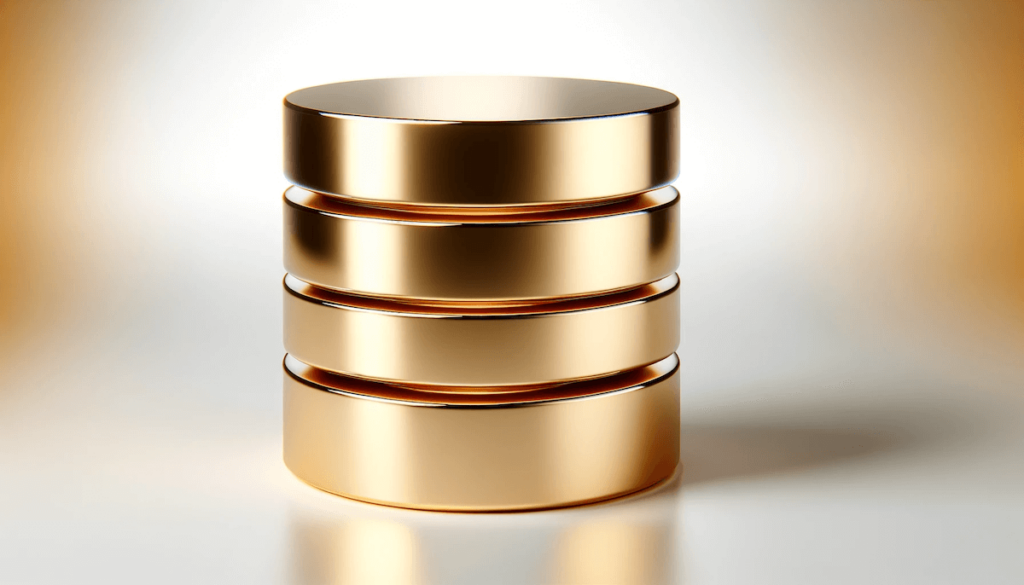
Another form of data quality is architectural — did the CRM implementation team set up the system to collect the types of information that will result in highly informed users and managers?
Striving for a gold standard of prospect, customer, vendor, and other data always pays off.
A 2022 survey conducted by Validity found that 44% of respondents estimated their company loses over 10% of annual revenue due to poor CRM data quality.
The same survey found that missing or incomplete data was the most significant impediment to respondents fully leveraging their CRM system.
Many organizations start with a pristine new CRM instance. But before long, CRM data decay sets in.
Both human input and data imports can contribute to the problem.
Thinking about data quality as early as possible in the CRM planning implementation process is essential.
Your organization will be better off if a proactive approach to data integrity and quality is taken rather than a reactive approach.
Who’s in Charge?
From a governance perspective, someone must be responsible for data quality and integrity.
CRM data won’t magically care for itself if no one is responsible for quality oversight.
CRM project managers need to anticipate potential causes of poor data quality and design the system for these.
Examples of Data Decay
Here are a few examples of how data quality issues can quickly arise.
Duplicate Records
Record duplication is one of the most common DQ issues in CRM.
There is often cross-table duplication. A person is a Lead and a Contact — or a Lead and multiple Contacts.
While technical rules in CRM can reduce duplicate creation, there is also an onus on users to check for existing records before creating new ones.
Obsolete Data
Appending ‘LEFT THE COMPANY’ to a Contact’s last name is not a quality approach for flagging a customer’s ex-employee.
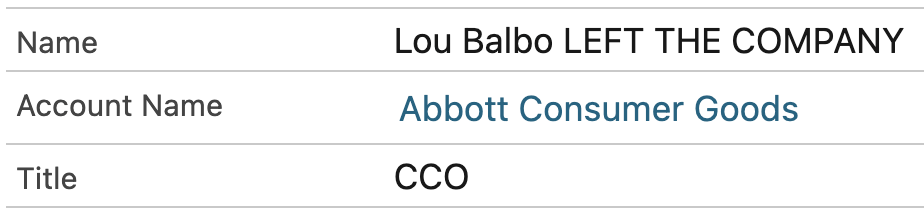
There should be a structured process for flagging defunct organizations and former employees.
This can be as simple as training CRM users to select a picklist value of ‘Inactive’ for obsolete accounts and contact records.
Global Accounts
Often, there’s no plan for managing global accounts with regional offices. For example, should every region have its own CRM account record in the format ‘Company Name – Region’?
Left to their own devices, users will collectively develop a hybrid of global account records — for some companies, there is one Account for the entire world. For others, there are multiple Accounts.
Picklist Values
No one ever asked for a report of all customers in the ‘Other’ industry or all Leads with a source of ‘Other.’
Picklists, a.k.a. dropdown values, should include all reasonable possibilities.
Values should be periodically assessed and updated based on changes to product and service offerings or the target audience.
Unverified Email Addresses
Invalid email addresses are an issue that can surface with mass list imports.
A domain’s sender reputation can be diminished when too many emails bounce.
Coupled with incomplete segmentation data, this issue can cause marketing campaigns to be delayed.
Unstructured Data
Freeform short and long text fields in a CRM system are okay for specific purposes.
However, searching and reporting are less effective when unstructured data fields are used for data that should be entered in structured data fields.
User ‘Creativity’
CRM users are sometimes very creative with data entry. They’ll enter two phone numbers into a phone field and label each.
Data fabrication can also occur. Users don’t have the information to add to a required field, so they’ll make something up.
Predicted Opportunity close dates are perhaps a CRM system’s greatest works of data fiction.
Strategies for Maintaining CRM Data Quality
For IT Project Managers, the following strategies are essential for preventing data decay and maximizing the value of CRM data to users and management.
System Configuration and Customization
Your CRM system must be designed to minimize the likelihood of data errors and ambiguity.
This means thinking through the implications of each added field.
Implement Validation Rules
Validation rules are essential for preventing erroneous data entry in specific fields.
Validation is essential enough to warrant examining each CRM vendor’s capabilities during a CRM selection process.
Configure Duplicate Rules Properly
Duplicate prevention is proactive. Deduplication is reactive.
If your CRM system has comprehensive duplicate avoidance features, be sure rules and actions are correctly set up.
Train Users
Despite all the ways technology can prevent CRM data decay, there’s a level of user responsibility for maintaining a gold data standard.
Users must be trained and periodically retrained on properly creating and updating CRM records.
It’s essential to communicate the ‘why’ of specific fields, as users may initially not see the value.
Users who provided pre-implementation input into the system may be more compliant with data entry standards due to the IKEA Effect.
As far as those fictional Opportunity close dates go, they need to be updated during sales meetings.
The quality and integrity of an organization’s CRM data can be a significant competitive advantage.
IT Project Managers must prioritize this aspect of CRM to maximize the success of their projects.